
Synthetic intelligence algorithms are increasingly remaining utilized in money products and services — but they arrive with some significant threats all around discrimination.
Sadik Demiroz | Photodisc | Getty Illustrations or photos
AMSTERDAM — Artificial intelligence has a racial bias difficulty.
From biometric identification systems that disproportionately misidentify the faces of Black men and women and minorities, to programs of voice recognition software that fail to distinguish voices with distinctive regional accents, AI has a whole lot to perform on when it will come to discrimination.
And the issue of amplifying current biases can be even a lot more significant when it comes to banking and money solutions.
Deloitte notes that AI techniques are in the end only as excellent as the details they’re given: Incomplete or unrepresentative datasets could restrict AI’s objectivity, although biases in growth groups that educate such units could perpetuate that cycle of bias.
A.I. can be dumb
Nabil Manji, head of crypto and World wide web3 at Worldpay by FIS, mentioned a key factor to fully grasp about AI products and solutions is that the energy of the know-how depends a large amount on the resource substance employed to train it.
“The matter about how superior an AI solution is, you will find variety of two variables,” Manji informed CNBC in an job interview. “A single is the info it has access to, and second is how great the massive language model is. That is why the data aspect, you see companies like Reddit and other individuals, they’ve occur out publicly and stated we’re not likely to allow organizations to scrape our knowledge, you happen to be going to have to fork out us for that.”
As for financial companies, Manji explained a good deal of the backend details systems are fragmented in diverse languages and formats.
“None of it is consolidated or harmonized,” he added. “That is likely to result in AI-pushed products and solutions to be a good deal significantly less helpful in economic solutions than it may be in other verticals or other businesses where by they have uniformity and a lot more modern day units or access to facts.”

Manji recommended that blockchain, or distributed ledger technological innovation, could provide as a way to get a clearer look at of the disparate data tucked absent in the cluttered devices of classic banking companies.
Nonetheless, he added that financial institutions — being the closely regulated, gradual-moving establishments that they are — are unlikely to transfer with the similar speed as their much more nimble tech counterparts in adopting new AI applications.
“You have received Microsoft and Google, who like about the last decade or two have been observed as driving innovation. They can not keep up with that velocity. And then you imagine about monetary services. Banking institutions are not acknowledged for getting quick,” Manji explained.
Banking’s A.I. trouble
Rumman Chowdhury, Twitter’s previous head of equipment studying ethics, transparency and accountability, said that lending is a primary instance of how an AI system’s bias versus marginalized communities can rear its head.
“Algorithmic discrimination is truly very tangible in lending,” Chowdhury reported on a panel at Revenue20/20 in Amsterdam. “Chicago experienced a historical past of virtually denying those [loans] to mainly Black neighborhoods.”
In the 1930s, Chicago was known for the discriminatory apply of “redlining,” in which the creditworthiness of houses was seriously determined by the racial demographics of a offered community.
“There would be a large map on the wall of all the districts in Chicago, and they would draw purple lines through all of the districts that were principally African American, and not give them financial loans,” she added.
“Rapid ahead a several a long time later, and you are producing algorithms to establish the riskiness of distinctive districts and folks. And although you might not incorporate the details issue of someone’s race, it is implicitly picked up.”
Without a doubt, Angle Bush, founder of Black Girls in Artificial Intelligence, an group aiming to empower Black women in the AI sector, tells CNBC that when AI programs are exclusively applied for mortgage acceptance selections, she has uncovered that there is a risk of replicating existing biases existing in historic info used to teach the algorithms.
“This can consequence in automated mortgage denials for people today from marginalized communities, reinforcing racial or gender disparities,” Bush additional.
“It is very important for banking institutions to accept that employing AI as a resolution may possibly inadvertently perpetuate discrimination,” she mentioned.
Frost Li, a developer who has been doing work in AI and equipment discovering for around a decade, advised CNBC that the “personalization” dimension of AI integration can also be problematic.
“What is attention-grabbing in AI is how we choose the ‘core features’ for education,” stated Li, who launched and operates Loup, a enterprise that assists on the internet retailers combine AI into their platforms. “Often, we decide on options unrelated to the final results we want to predict.”
When AI is used to banking, Li suggests, it is tougher to identify the “perpetrator” in biases when all the things is convoluted in the calculation.
“A fantastic example is how numerous fintech startups are specially for foreigners, simply because a Tokyo University graduate will never be ready to get any credit score cards even if he performs at Google nevertheless a human being can effortlessly get a person from group college or university credit rating union because bankers know the nearby schools much better,” Li additional.
Generative AI is not ordinarily applied for developing credit rating scores or in the hazard-scoring of buyers.
“That is not what the tool was developed for,” explained Niklas Guske, chief running officer at Taktile, a startup that aids fintechs automate choice-building.
Rather, Guske mentioned the most impressive purposes are in pre-processing unstructured knowledge this sort of as text files — like classifying transactions.
“Those indicators can then be fed into a a lot more classic underwriting product,” stated Guske. “As a result, Generative AI will make improvements to the underlying facts excellent for this kind of conclusions alternatively than switch frequent scoring processes.”
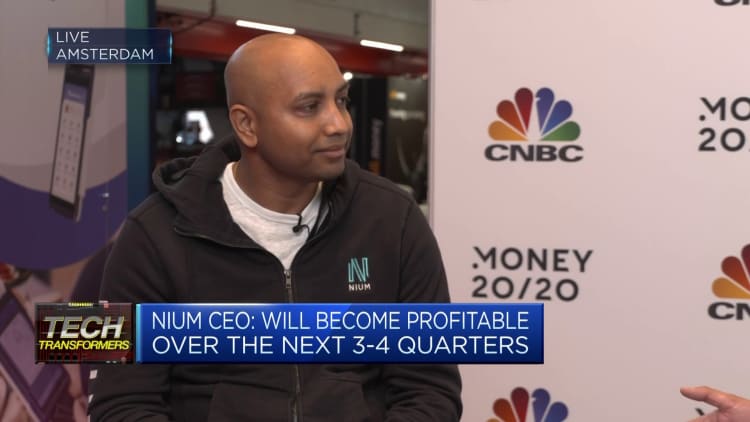
But it’s also challenging to prove. Apple and Goldman Sachs, for instance, ended up accused of providing women decrease limitations for the Apple Card. But these promises were dismissed by the New York Division of Money Expert services following the regulator uncovered no evidence of discrimination primarily based on intercourse.
The dilemma, in accordance to Kim Smouter, director of anti-racism group European Network Towards Racism, is that it can be challenging to substantiate no matter if AI-dependent discrimination has really taken position.
“A person of the complications in the mass deployment of AI,” he reported, “is the opacity in how these choices arrive about and what redress mechanisms exist have been a racialized unique to even see that there is discrimination.”
“Folks have minimal awareness of how AI techniques operate and that their particular person circumstance could, in point, be the tip of a techniques-huge iceberg. Appropriately, it’s also tricky to detect specific circumstances wherever matters have long gone erroneous,” he extra.
Smouter cited the illustration of the Dutch youngster welfare scandal, in which 1000’s of reward statements were being wrongfully accused of staying fraudulent. The Dutch governing administration was pressured to resign soon after a 2020 report uncovered that victims had been “dealt with with an institutional bias.”
This, Smouter explained, “demonstrates how promptly these types of disfunctions can distribute and how hard it is to confirm them and get redress after they are uncovered and in the meantime sizeable, normally irreversible injury is completed.”
Policing A.I.’s biases
Chowdhury claims there is a require for a international regulatory overall body, like the United Nations, to tackle some of the dangers bordering AI.
Though AI has proven to be an progressive resource, some technologists and ethicists have expressed doubts about the technology’s moral and ethical soundness. Amid the top anxieties marketplace insiders expressed are misinformation racial and gender bias embedded in AI algorithms and “hallucinations” created by ChatGPT-like applications.
“I fret really a little bit that, due to generative AI, we are coming into this write-up-reality earth exactly where nothing at all we see on the net is trustworthy — not any of the text, not any of the movie, not any of the audio, but then how do we get our information and facts? And how do we make sure that information has a substantial total of integrity?” Chowdhury claimed.
Now is the time for significant regulation of AI to arrive into drive — but realizing the volume of time it will take regulatory proposals like the European Union’s AI Act to consider impact, some are involved this won’t happen fast plenty of.
“We call upon more transparency and accountability of algorithms and how they operate and a layman’s declaration that enables persons who are not AI gurus to decide for them selves, evidence of testing and publication of final results, unbiased grievances process, periodic audits and reporting, involvement of racialized communities when tech is becoming created and regarded as for deployment,” Smouter claimed.
The AI Act, the to start with regulatory framework of its variety, has incorporated a elementary legal rights approach and principles like redress, in accordance to Smouter, incorporating that the regulation will be enforced in about two yrs.
“It would be excellent if this time period can be shortened to make confident transparency and accountability are in the core of innovation,” he explained.
